Feb 11, 2025
Abstract:This research explores the opportunities of Generative AI (GenAI) in the realm of higher education through the design and development of a multimodal chatbot for an undergraduate course. Leveraging the ChatGPT API for nuanced text-based interactions and Google Bard for advanced image analysis and diagram-to-code conversions, we showcase the potential of GenAI in addressing a broad spectrum of educational queries. Additionally, the chatbot presents a file-based analyser designed for educators, offering deep insights into student feedback via sentiment and emotion analysis, and summarising course evaluations with key metrics. These combinations highlight the crucial role of multimodal conversational AI in enhancing teaching and learning processes, promising significant advancements in educational adaptability, engagement, and feedback analysis. By demonstrating a practical web application, this research underlines the imperative for integrating GenAI technologies to foster more dynamic and responsive educational environments, ultimately contributing to improved educational outcomes and pedagogical strategies.
* 9 pages, 4 figures, accepted and presented in the 2025 6th
International Conference on Advances in Education and Information Technology
(AEIT)
Via
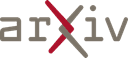